Parchment Student Choice College Rankings 2021
College rankings based on actual student enrollment decisions
Tens of thousands of students have contributed to a database of over 3,673,831 acceptances at hundreds of colleges in the US. Using a formula similar to the one used to rank chess masters, Parchment helps decipher the meaning of those student choices.
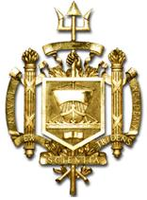
- Nearby Cities: Washington, Baltimore, Northern Virginia
- Enrolled Students: 5,660
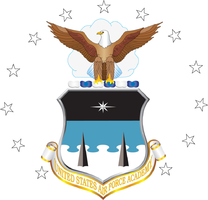
- Nearby Cities: Colorado Springs
- Enrolled Students: 5,062
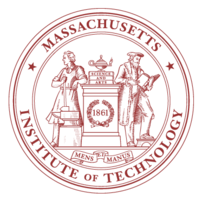
- Nearby Cities: Boston, Worcester, Manchester
- Enrolled Students: 4,504
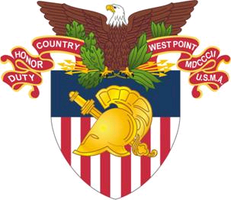
- Nearby Cities: New York, Newark, Bridgeport
- Enrolled Students: 4,536
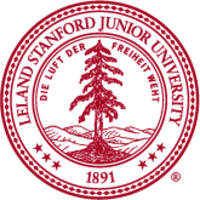
- Nearby Cities: San Jose, San Francisco, Oakland
- Enrolled Students: 7,412
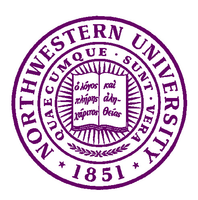
- Nearby Cities: Chicago, Naperville, Michigan City
- Enrolled Students: 8,677

- Nearby Cities: Kansas City, Overland Park, Kansas City
- Enrolled Students: 6,686
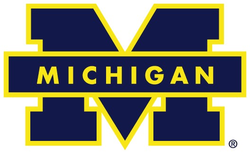
- Nearby Cities: Detroit, Warren, Flint
- Enrolled Students: 27,880
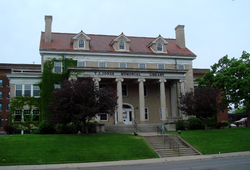
- Nearby Cities: Minneapolis, St. Paul, St. Cloud
- Enrolled Students: 1,042
How It Works
When students are admitted to multiple colleges, they must choose just one to attend; this reveals their preference for the chosen school compared to the other schools that admitted the student but were not chosen. After observing enough of these decisions, we can rank the colleges based on the students' revealed preferences.
To start, we assign all of the colleges the same number of points (1500), because we do not know which colleges are preferred over others. For each student, we then match up the colleges that accepted them to the college that they chose. If a student was accepted to 2 colleges, then there is 1 matchup (1 chosen school vs 1 not-chosen school). If a student was accepted to 3 colleges, then there are 2 matchups (1 chosen school vs 2 not-chosen schools). So for every student who was admitted to N colleges, there are N-1 matchups.
Matchups are scored based on expectation. At the beginning, all schools have the same score, so we expect that all schools have an equal chance of winning a matchup. Quickly, our expectations change. We see that Berkeley rapidly gains points, for example. Our Elo-based system awards more points for unexpected victories against a college with a higher score, an average number of points for victories against another college with a similar score, and fewer points for expected victories against a school with fewer points. In fact, the number of points awarded to the chosen school (and taken from the not-chosen school) is determined by a formula that takes into account the current score of both schools. To use Berkeley as an example again, when Berkeley is chosen over a school with a similar amount of points (such as UCLA), it earns more points than when it is chosen over a school with fewer points. If a student were to choose to attend a university with far fewer points than Berkeley, that university would gain many more points from that victory than Berkeley would have if the student had chosen Berkeley instead.
At the end of this process, we rank the colleges by the number of points that they have. There is a formula that converts these points into the probability that a student will attend one college over another, so we can actually look at the output and say, "There is an 84% chance that a student accepted to Berkeley and Texas A&M would choose Berkeley." And in that way, our ranking system directly translates into a tangible, meaningful summary of the actual preferences expressed by tens of thousands of students through the decisions they made.
Advanced Details
After we work through all of the matchups, we’re not quite done. Our approach is path dependent. What if, by chance, we first came upon all of the students who chose Berkeley over other colleges, and then later we came upon all of the students who chose other colleges over Berkeley? At the beginning, Berkeley would accumulate points (perhaps more than it should, on average). But at the end, Berkeley would be donating more points than it should to the colleges that its applicants chose to attend instead.
The solution is to order the matchups by admissions cycle, but to randomize them within each admissions cycle (in essence, we perform a Monte Carlo simulation). We start with the oldest data that we use (from 2009). We process all of the matchups from that year. Then, we randomize the order and process them all again. (For each round of reprocessing, we sample the matchups without replacement.) We do this 10,000 times. Then, we take those scores as the starting point for the 2010 matchups, and so on, until we reach the latest year from which we have admissions data and produce our final rankings. We go in order of admissions cycle because the past informs the future (by the time 2010 rolls around, we could have observed all of the 2009 data). But within each admissions cycle, all matchups can be considered to be simultaneous. Information essentially does not leak within a cycle, which is why there is no true order to the admissions decisions within an admissions cycle, and we must repeatedly randomize the order of the matchups in order to get stable estimates of the results of that cycle.